Multiscale Modeling of Bioreactors
Simon Kahan, Boris Aguilar, Jiajia Chen, Jaro Camphuijsen, Fernando J. Cantarero Rivera, Greg Potter, Chris Clark
Funded by The Good Food Institute
tHE GOAL
Integrating agent-based modeling with computational fluid dynamics to predict biomass outcomes in bioreactors to form a generally applicable computational pipeline. We apply this pipeline to stirred tank bioreactors and recapitulate the biomass production as a function of impeller speed presented in the literature. We also apply this pipeline to model the behavior of cells that are seeded in an IBIDI flow chamber, a controlled system that will generate crucial experimental data for the development of models of cell behavior under shear stresses induced by fluids.
The bioreactor modeling framework as developed in this project, combining agent based modeling with computational fluid dynamics to simulate cell experience and behavior.
Fluid dynamics of various bioreactor designs
Fluid dynamics of various bioreactor designs were simulated using Random Averaged Navier Stokes with a kappa-epsilon turbulence model. Designs included the simple stirred tank bioreactor, a more advanced stirred tank design, the IBIDI flow chamber, a rocking bed bioreactor and a stacked plate bioreactor.
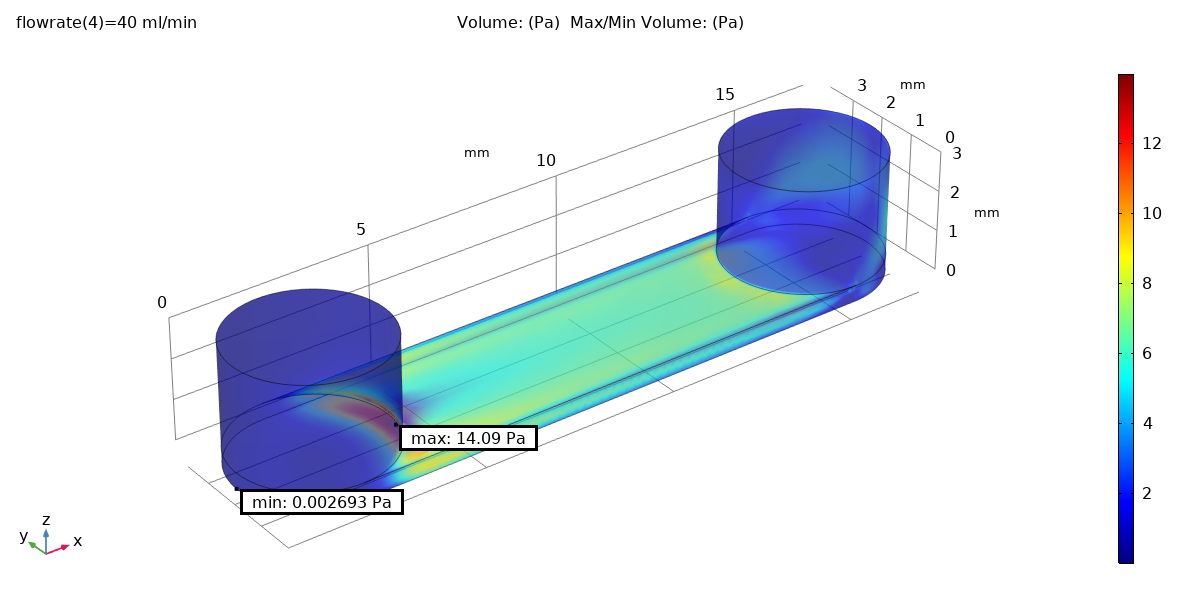
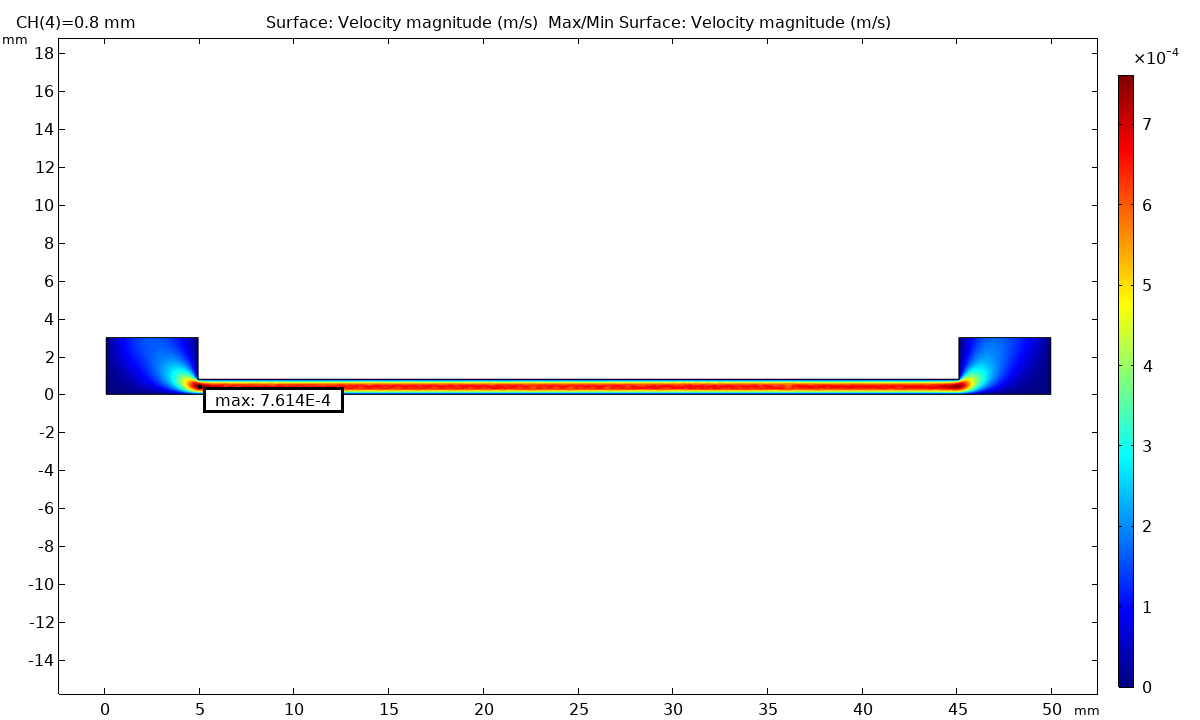
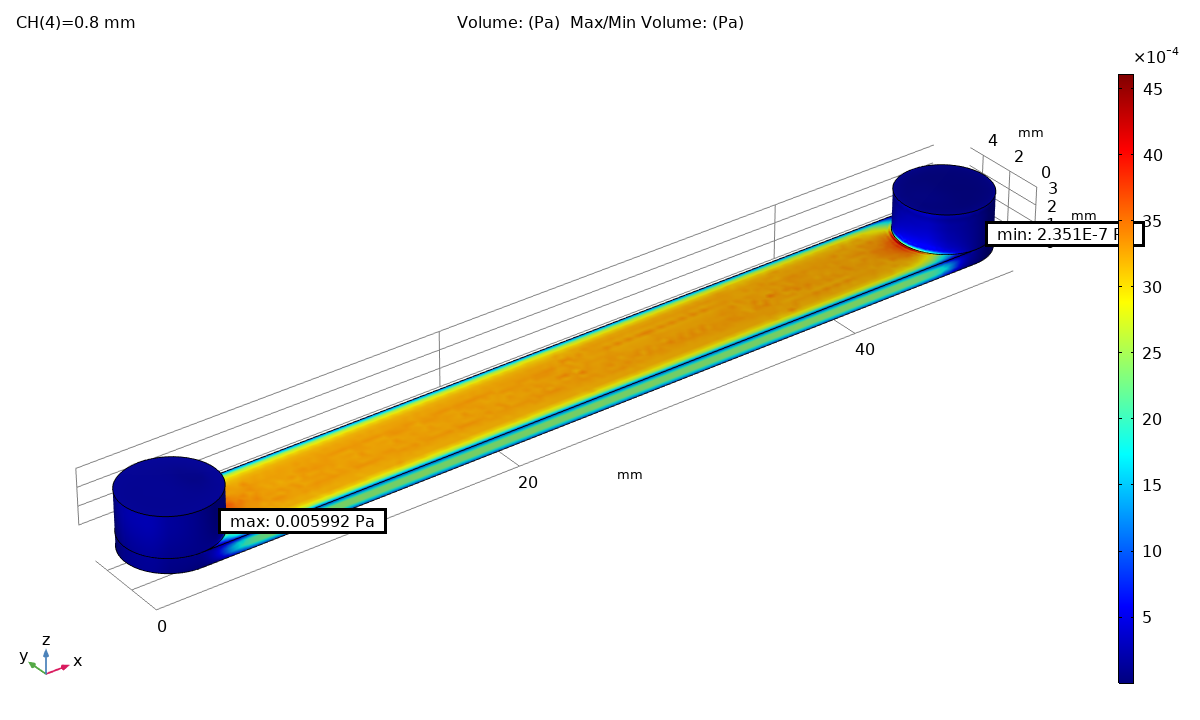
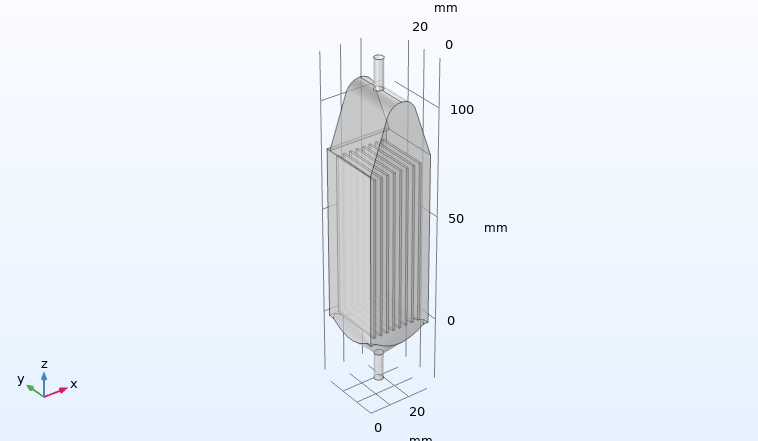
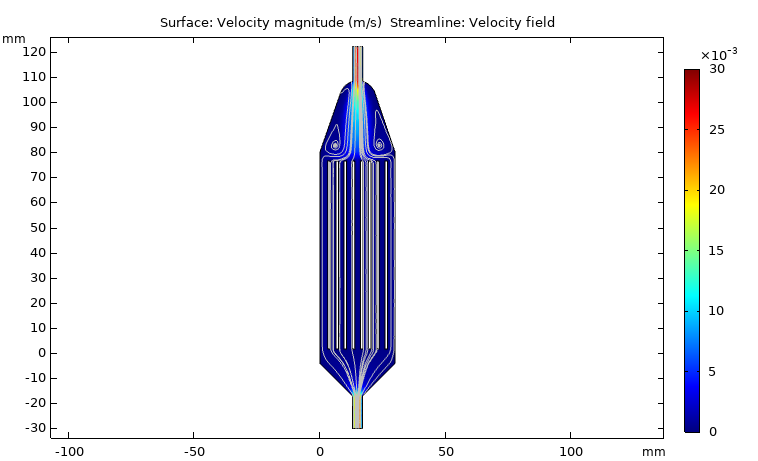
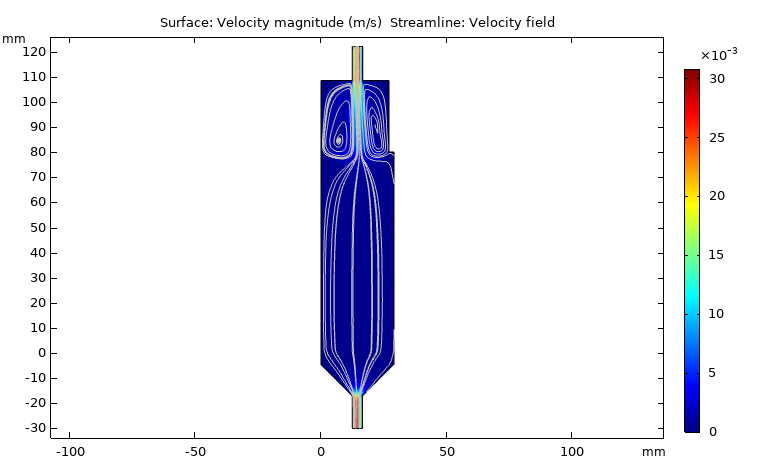
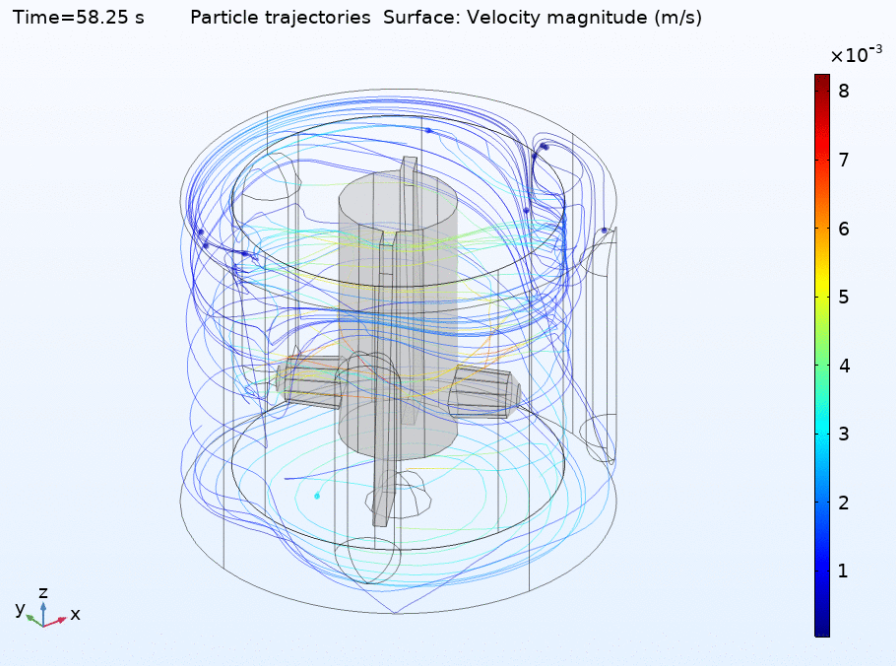
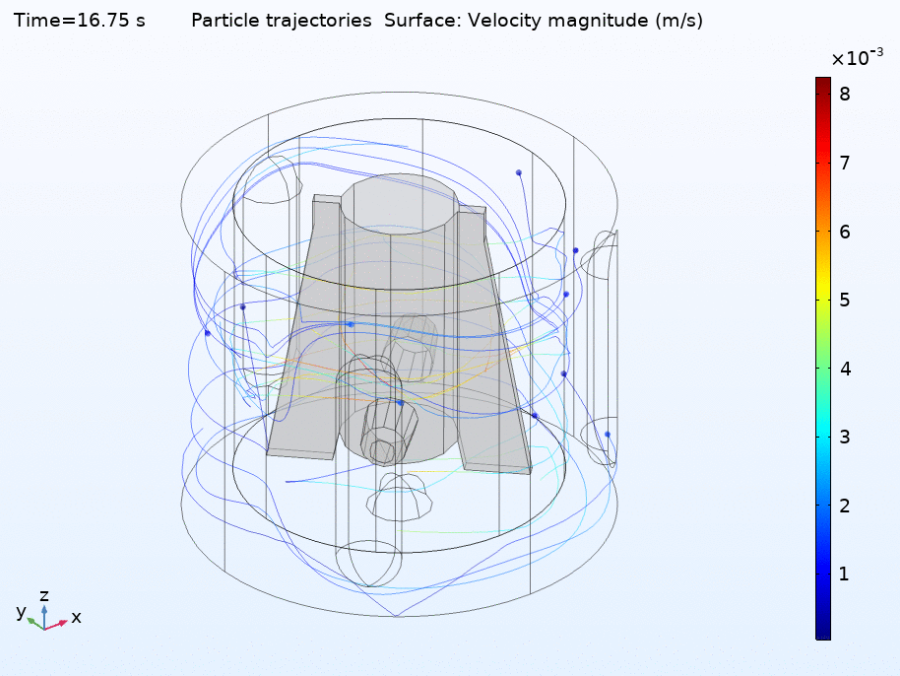
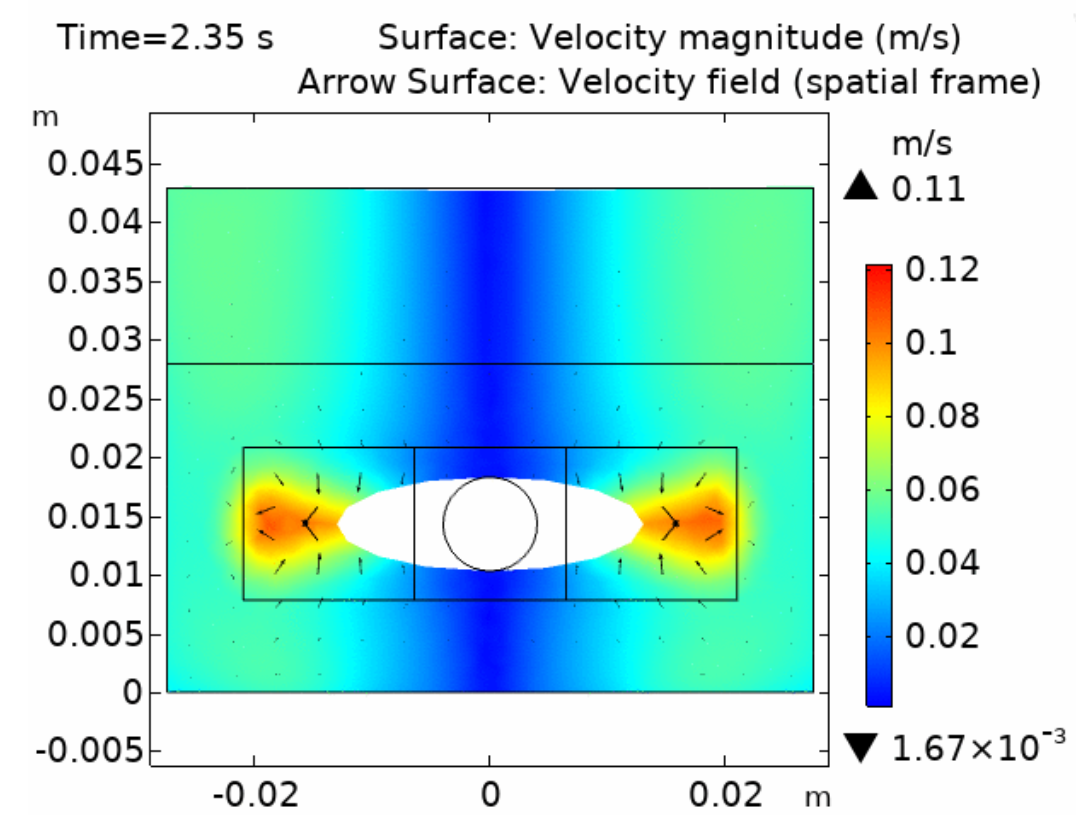
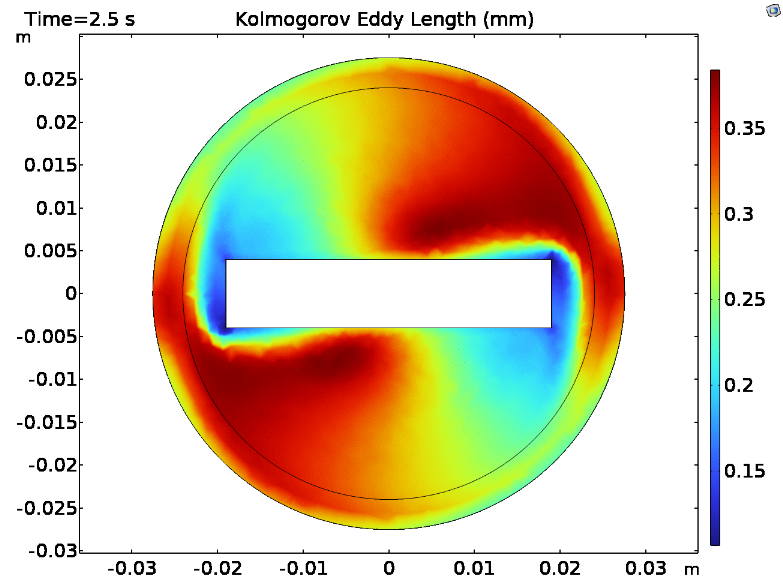
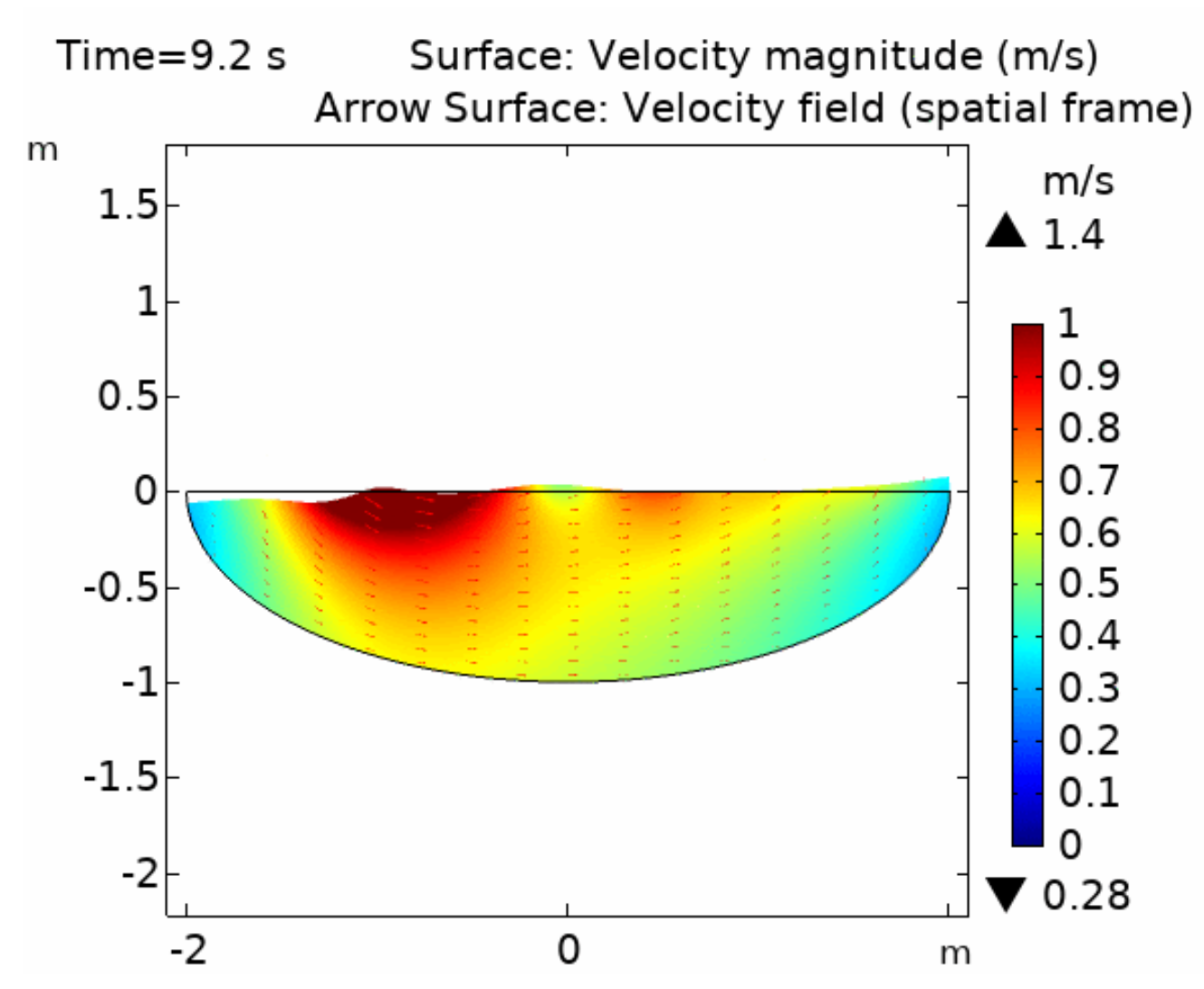
Results
An important output of the combined model was the cell count of the cells in the stirred tank bioreactor. We analyzed the cell counts for four different states of the cell model: alive, attached (to microcarrier), dying and dead. An analysis of the system with different cell model parameters and stir speeds, showed us the ability of this model to reproduce a great variety of behavior.
Another important output was the microcarrier only simulation that gave the distribution of microcarriers over the reactor in time. This showed an increasing density at certain “hot spots” which is most likely an artifact of the simplified coupling between the CFD and particle model. A special feature of the combined modeling approach is that the cell experience can be tracked on high resolution per single cell or group of cells. Due to the inaccuracies upstream in the modeling pipeline we did not analyze the cell experience for our simulations. However in principle this approach will enable to analyze in high detail in which parts of the reactor and at which moment in time, cells will experience high amounts of physical or other stress. For a complete summary of the results you can read our project paper.
The elementary effects sensitivity analysis of the stirred tank bioreactor model for various input parameter settings of the agent based model shows high variation in the sensitivity for most parameters.
Sensitivity analysis
To assess the consistency of our modeling runs and test for which inputs the model outcome would be most sensitive, we performed a sensitivity analysis according to the Elementary Effects method of Morris et al. (1991). From this analysis it became clear that our model was very sensitive to the various inputs, but even more due to the stochastic effects of the fluid dynamics submodel. The latter can be tackled by running more (or higher density of particles) simulations per input parameter set and analyze the resulting stochastic distributions.